Data integration technologies have evolved tremendously over the years. Manual processes and custom scripts marked the early days of data integration to move data between systems. Unfortunately, this approach was tedious, time-consuming, and error-prone. As such, data integration solutions evolved to help streamline the data integration process and other common business processes.
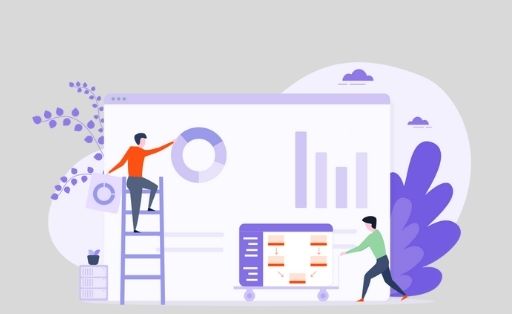
Contents
ESB Integration Era
The ESB Integration Era was a time when data integration evolved rapidly. The days of point-to-point integration were fading, and ESBs were becoming the de facto standard for integrating systems. ESBs offered a more centralized and automated way to integrate systems, and they could handle a much higher volume of data than point-to-point integrations.
The ESB Integration Era was a remarkable transformation for the data integration industry. ESBs were becoming the standard for data integration, and they were offering businesses a more efficient and more scalable way to integrate their systems. The future of data integration and application integration is looking bright, and the ESB Integration Era is paving the way for even greater innovation and progress.
Point-to-Point Integration Era
The early days of data integration were focused on point-to-point integration, where data was manually copied from one system to another. This was a tedious and time-consuming process, and it was challenging to keep the data in sync between the systems.
In the late 1990s, the first data integration tools began to emerge. These tools automated copying data from different systems, making it easier and faster to integrate the data pipeline. However, the early data integration tools were limited in their capabilities. For example, they could only copy data from one system to another and could not handle complex data transformations or large-scale data warehouse needs.
In the early 2000s, the first ETL (extract, transform, load) tools emerged. These tools provided more sophisticated capabilities, including handling complex data transformations. The early ETL tools were expensive and difficult to use, but they quickly became the standard for application integration. The late 2000s saw the emergence of cloud-based data integration tools. These tools provided a more affordable and easier-to-use alternative to the traditional ETL tools.
The early 2010s saw the emergence of predictive data quality tools, which provided the ability to handle large volumes of data. The current era of data integration is focused on big data and the cloud. Big data tools offer the ability to handle large volumes of data, and cloud-based data integration tools provide the ability to easily and quickly integrate data from multiple sources.
Data lakes and data hubs have become popular
Data integration has come a long way over the years. Initially, companies integrated data into a very manual process. This involved extracting data from different sources, consolidating it into a single format, and then loading it into a database.
In recent years, data lakes and data hubs have become popular. A data lake is a repository for storing all of your organization’s data in its original format. This can include both structured and unstructured data. A data hub is a centralized repository for data from multiple sources. You can use it to consolidate data from different data lakes or data warehouses.
Both data lakes and data hubs make modern data integration much easier and faster. They allow you to consolidate data from multiple sources into a single format. This makes it easier to analyze and report on the data.
Businesses move from centralized to decentralized data architectures
As companies have become more reliant on data, the need for efficient data integration project types has grown. Companies relied on centralized data architectures in the early days of data integration. This approach required all data to be funneled through a single system, which could be difficult and expensive to scale.
With the rise of big data and the internet of things, businesses began to move to decentralized data architectures. This approach allows enterprises to store data in multiple locations, making it easier to scale and manage. Decentralized data architectures also make it easier to process data in real-time, essential for businesses that rely on big data. With an appropriate data integration project, you can empower your business.